DocentPro is building an AI travel platform that helps travelers figure out where to go, what to do, and how to plan.
People love using ChatGPT and Perplexity for trip research - and they’re great at surfacing ideas. But to DocentPro, travel isn’t just about a research – it’s about creating a streamlined experience from discovery to planning to booking. These tools often stop short. You still end up mapping routes, comparing ratings, scanning reviews, and bouncing between apps to make it all work.
To solve that, they built a modular multi-agent system using LangGraph and LangSmith, blending the creativity of LLMs with the precision of deterministic logic, and designing reusable agents that work across trip planning and real-time conversation.
Building Modular Agents that Work Across the Stack
DocentPro broke down the travel planning agent into four domains: attractions, restaurants, hotels, and activities. Each of these is managed by its own agent, and each agent is designed to be modular and reusable. That means the Restaurant Research Agent, for example, isn't just used in their trip planner to suggest meals for each day. The same agent is also called by their chat assistant when a user asks, "Where’s a good place to eat nearby?"
This approach helps the DocentPro team avoid duplicating logic, keep things consistent, and make their agents easier to test and improve. With LangGraph, each part of the system is composed as a clear, traceable node - and with LangSmith, they can debug and refine behavior step-by-step. DocentPro thinks of this as a small but important step toward more collaborative AI - where agents don’t just operate in isolation, but work together across different workflows.
Balancing LLM Flexibility with Deterministic Control
While LLMs are great at coming up with interesting ideas - like suggesting popular local spots - they don’t always stick to reality. Furthermore, while LLMs are great at suggesting interesting places, especially well-known spots, they often build itineraries based solely on what they “know,” not how people actually move. The result? Plans that zigzag across the regions in a day without considering realistic routes.
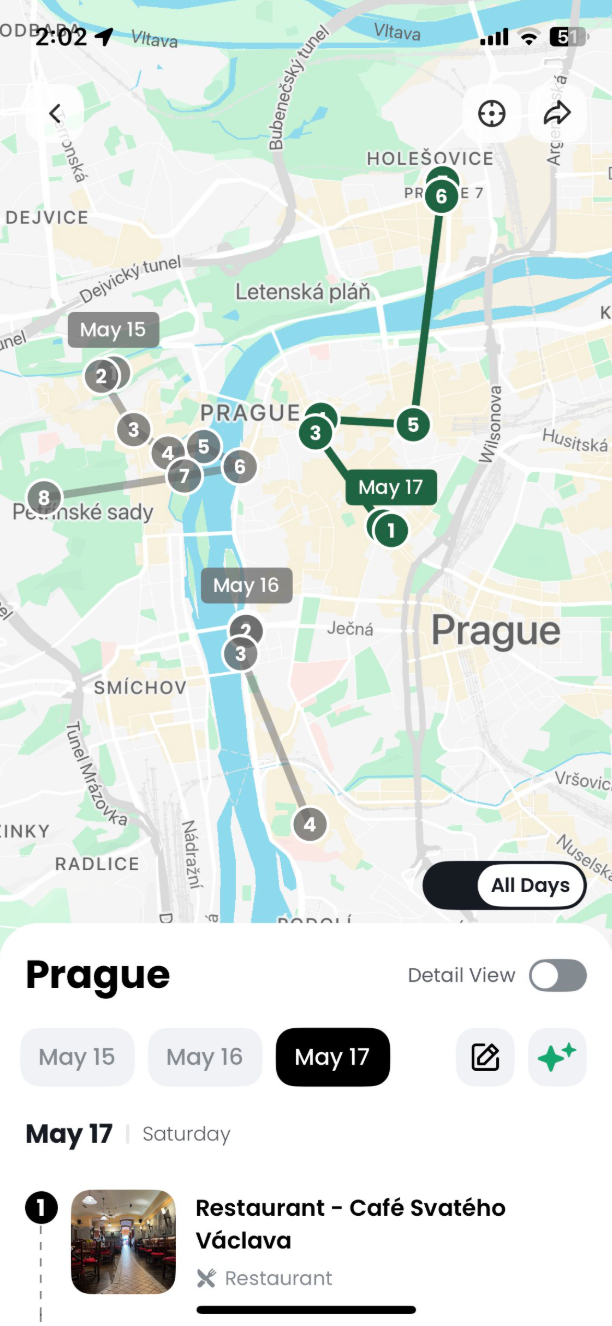
That’s why DocentPro built guardrails into the system to keep outputs grounded. This involved:
- K-Means clustering to group points of interest by geography
- Route reordering to minimize unnecessary travel
- Filtering out hallucinated or closed places
- LLM-generated explanations for every recommendation
This hybrid approach helps DocentPro strike the right balance between helpful suggestions and practical plans that users can actually follow.
Observability and Debugging with LangSmith
LangSmith has been essential in helping DocentPro make their system reliable. They use it to:
- Trace and monitor every LangGraph run
- Quickly inspect where things go wrong (or right)
- Understand how users are interacting with our agents
- Replay sessions to iterate faster and improve behavior
It’s especially helpful in a multi-agent system, where it’s easy to lose track of who’s doing what. With LangSmith, the DocentPro team always has visibility into the decision-making process.
Adding support for Audio Guides in 12 Languages
One of the earlier features DocentPro built was an on-demand audio guide system for global attractions. They initially implemented it with a custom RAG pipeline - but as they added support for 12 languages and more locations, maintaining and scaling the system became painful.
As a result, DocentPro decided to port their architecture to LangGraph in just two days, using a map-reduce style workflow tailored for content generation. This means that:
- For each point of interest, they break it down into multiple topics (e.g. history, architecture, fun facts)
- Each topic is passed through a chain of agents: research ⇒ narrative generation(RAG) ⇒ translation ⇒ TTS(Text to Speech)
- Final outputs are then aggregated into structured, per-language audio playlists
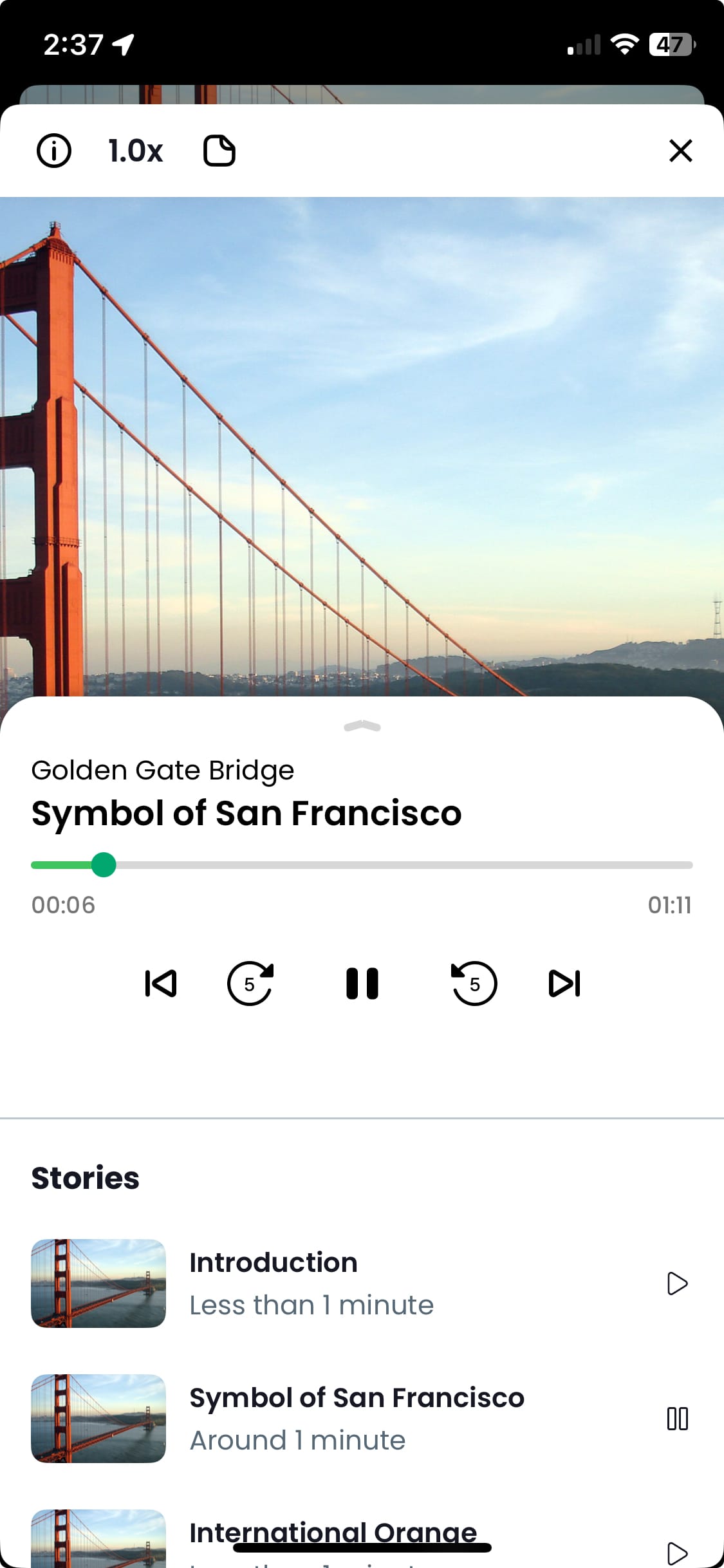
LangSmith helped DocentPro trace and debug the early runs, and now the system scales globally with minimal overhead.
The Result
DocentPro’s current system:
- Uses modular, domain-specific agents across trip planning and chat
- Combines deterministic algorithms with LLM-based reasoning
- Is fully traceable and improvable via LangSmith
- Powers AI itineraries and multilingual audio guides for travelers around the world
DocentPro is continuing to improve how their agents interact and how they bring structure to flexible travel - one itinerary at a time.